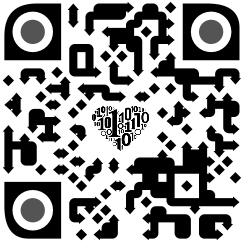
Naše nejvýznamnější odborné publikace
Medical Informatics - University of West Bohemia (CZE)
Web: https://mi.kiv.zcu.cz
Web: https://mi.kiv.zcu.cz
All 2018 2017 2016 2015 2014 2013 2012 2011
2018
BRŮHA, P., MOUČEK, R., VACEK, V., ŠNEJDAR, P., ČERNÁ, K., ŘEHOŘ, P. Collection of human reaction times and supporting health related data for analysis of cognitive and physical performance. Data in Brief, 2018, Volume 17, Issue April 2018, p. 469-511. ISSN: 2352-3409
Abstract: Smoking, excessive drinking, overeating and physical inactivity are well-established risk factors decreasing human physical performance. Moreover, epidemiological work has identified modifiable lifestyle factors, such as poor diet and physical and cognitive inactivity that are associated with the risk of reduced cognitive performance. Definition, collection and annotation of human reaction times and suitable health related data and metadata provides researchers with a necessary source for further analysis of human physical and cognitive performance. The collection of human reaction times and supporting health related data was obtained from two groups comprising together 349 people of all ages - the visitors of the Days of Science and Technology 2016 held on the Pilsen central square and members of the Mensa Czech Republic visiting the neuroinformatics lab at the University of West Bohemia. Each provided dataset contains a complete or partial set of data obtained from the following measurements: hands and legs reaction times, color vision, spirometry, electrocardiography, blood pressure, blood glucose, body proportions and flexibility. It also provides a sufficient set of metadata (age, gender and summary of the participant's current life style and health) to allow researchers to perform further analysis. This article has two main aims. The first aim is to provide a well annotated collection of human reaction times and health related data that is suitable for further analysis of lifestyle and human cognitive and physical performance. This data collection is complemented with a preliminarily statistical evaluation. The second aim is to present a procedure of efficient acquisition of human reaction times and supporting health related data in non-lab and lab conditions.
VAŘEKA, L., MAUTNER, P. Modifications of unsupervised neural networks for single trial P300 detection. Neural Network World, 2018, Volume 28, Issue 1, p. 1-16. ISSN: 1210-0552
Abstract: P300 brain-computer interfaces (BCIs) have been gaining attention in recent years. To achieve good performance and accuracy, it is necessary to optimize both feature extraction and classification algorithms. This article aims at verifying whether supervised learning models based on self-organizing maps (SOM) or adaptive resonance theory (ART) can be useful for this task. For feature extraction, the state-of-the-art Windowed means paradigm was used. For classification, proposed classifiers were compared with state-of-the-art classifiers used in BCI research, such as Bayesian Linear Discriminant Analysis, or shrinkage LDA. Publicly available datasets from 15 healthy subjects were used for the experiments. The results indicated that SOM-based models yield better results than ART-based models. The best performance was achieved by the LASSO model that was comparable to state-of-the-art BCI classifiers. Further possibilities for improvements are discussed